Our view at Stack - Simplify web development with Webflow, reduce costs, and deliver professional results. No-code, responsive, and SEO-friendly. Explore your creative potential!
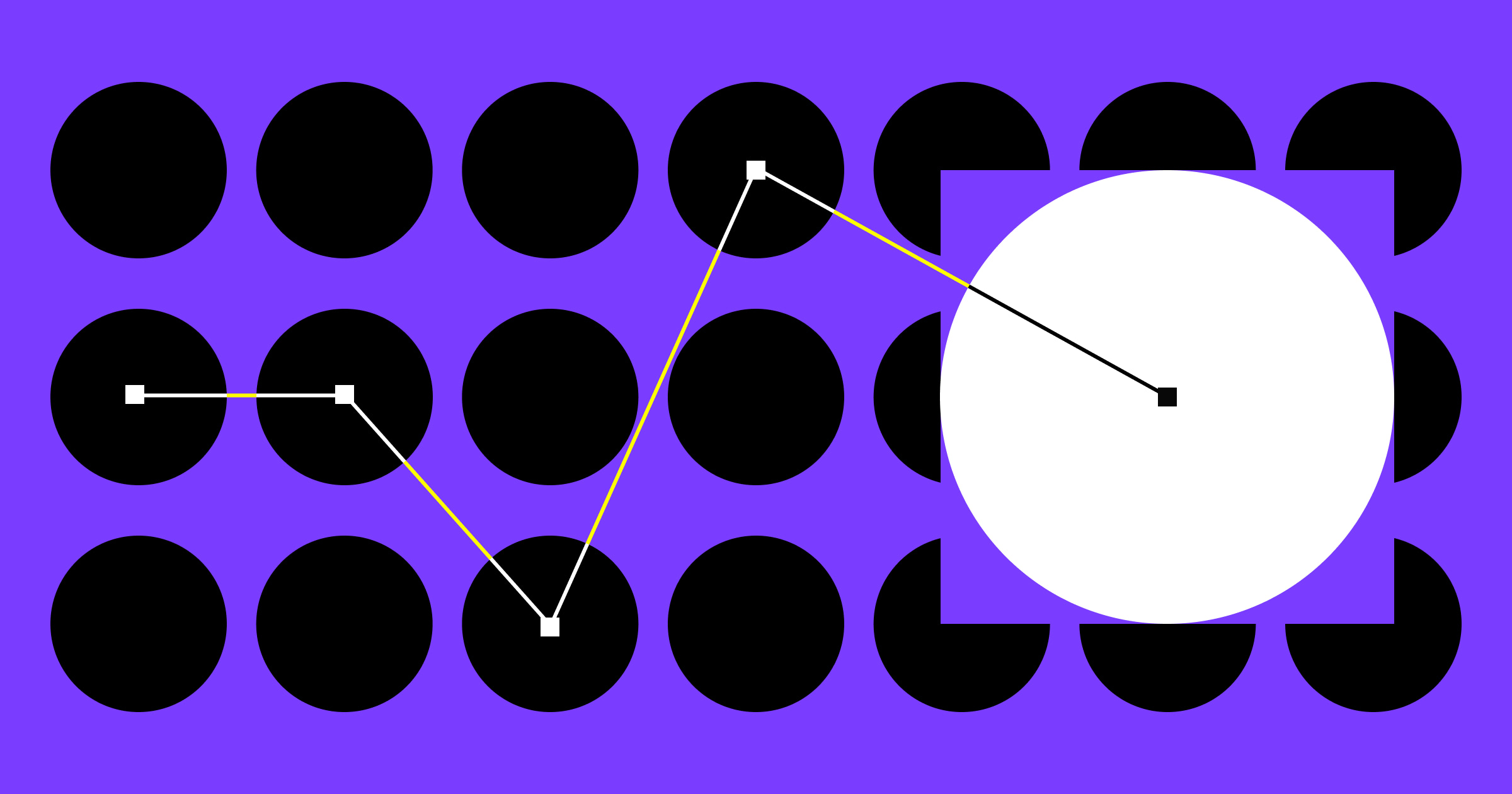
AI is pushing data analysis into new territories.
AI excels at digesting and extracting critical insights from massive datasets. Pre-AI, data professionals performed this work, spending immense amounts of time collecting, refining, and interpreting information. But with AI’s advancing capabilities, you can automate data analysis processes that would require dozens or hundreds of hours to complete manually.
That’s what makes AI and data analytics such a potent combination. AI helps analysts bypass tedious data collection and interpretation, skipping directly to managing, validating, and using data to craft accurate reports for businesses.
An overview of AI in data analytics
Applying AI to data analysis is a powerful way to quickly and accurately sift through massive datasets. This approach leans into what AI does best: detecting patterns and using them to predict outcomes.
AI-powered analytics tools combine machine learning (ML) and data analytics for faster processing than humans can perform. They excel at drawing straightforward connections and interesting conclusions humans might miss, such as trends with unintuitive correlations or extremely long-term cycles. And they can even help write SEO content that drives more traffic to your website.
When you pair an AI learning algorithm with your data analysis efforts, you can expect the following benefits.
Speed and efficiency
Analysts can feed an AI analysis system their data and turn to other tasks while it processes. This efficiency offers new freedom to data professionals — it assumes tedious tasks and lets employees focus on more meaningful work: interpreting data to discover insights.
Validation
Data validation is critical to finalizing an analysis, and AI supports this crucial step. After discovering a combination of data points to include in a report, analysts can use AI to instantly call up those points for review. This automation dramatically reduces the time it takes to write SQL queries and search through reports.
Ease of use
Most AI analysis tools offer natural language processing (NLP), which lets analysts use natural language to interact with their data. For example, you might write, “Search this dataset for the five most purchased products,” or “How have in-person and online sales changed over the last two years?”
This simplicity offers your team more freedom in how you interact with your database. It also lowers the barrier to entry for new analysts by fast-tracking much of the learning curve.
3 types of AI used in data analytics
You can distinguish different AI types by their relative complexity. The more sophisticated an AI tool is, the more capable it is of finding subtle correlations.
Here are three types of AI commonly used in data analysis.
1. Machine learning
ML algorithms are trained, meaning developers feed them vast amounts of data and instruct them to extract conclusions. From this process, the algorithms “learn” what to look for in new datasets.
When you feed information to a trained ML algorithm, you don’t need to provide it with any instruction. The algorithm uses what it’s learned to search your data and create conclusions. Along the way, it continues to refine that knowledge base for improved data analysis capabilities.
2. Neural networks
More advanced than ML’s system of overlapping processes, neural networks aim to simulate the human brain. They do so using interconnected processes representing nodes that pass information back and forth for analysis. This process enables them to discover connections an ML algorithm’s more straightforward process might miss.
3. Deep learning
The most advanced of these AI types, deep learning involves training a neural network with a massive dataset, teaching it to recognize things and interpret patterns. This technology is what self-driving cars use to identify other vehicles on the road and evaluate changing conditions. It’s equally effective at discovering patterns in data that a human might overlook.
How AI can improve your data analytics process
AI makes handling and interpreting data easier than ever because it seamlessly fits into many analytics processes. Here are a few suggestions for where to integrate AI into business data strategies.
Data cleaning
The first step in analyzing a database is checking it for errors. Analysts spend significant time scanning through hundreds of lines in a spreadsheet or writing detailed SQL queries to surface such mistakes. Thankfully, AI can perform this task much faster. You simply feed the AI your database and use NLP to form a query, such as “Check this database for missing information and errors.”
Data analysis
AI eases the burden of analysis for data professionals without replacing the need for a human eye. You can use AI to search the data, but it’s up to you to determine what’s important. For example, if you feed your data to a deep learning algorithm, it’ll churn out dozens of trends, predictions, and correlations you might never have found. But it’s up to you to sift through these results and surface the most relevant, informative bits for your business.
Data visualization
Another common task is creating visuals for the information you analyze. You might use tools like Zoho and Tableau to do this, which increasingly feature AI-generated visualizations to quicken the process. For example, Tableau Pulse uses AI to generate customizable data visualizations based on predefined KPIs.
Predictive analysis
AI excels at discovering intricate trends in data and mapping them out over a timeline — which requires simple math to plot hundreds or thousands of points over time. AI can process these trends significantly faster than a human can, and it can identify correlations to generate intricate forecasts.
One example of AI predictive analysis is Life2Vec, a powerful tool that uses basic information about your age, weight, and exercise habits to predict your potential lifespan.
Industry use cases of AI data analytics
AI has quickly permeated every industry thanks to its uniquely versatile capabilities. Here are some examples of how AI supports industry processes, from basic data collection to complex analysis.
Healthcare
The healthcare industry generates enormous datasets every day, from X-ray and MRI images to BMI and blood test measurements. AI algorithms consume this data to find connections between data points sooner than a medical professional might. These advancements help with early detection of cancer, diabetes, and Alzheimer’s, improving healthcare outcomes and potentially saving lives.
Finance
With its convoluted regulations and mind-boggling complexity, the financial industry benefits from any advancement in AI technology that simplifies data analysis. AI is an incredible tool financiers can use to advance their portfolios through speculating on stock prices and analyzing economic conditions. For example, the financial platform OneStream uses ML to find signals in the economic landscape that indicate a financial strategy’s potential success or failure.
Marketing and customer insights
Interpreting critical user data helps businesses optimize products, services, and marketing campaigns for certain goals, such as increasing conversions or driving engagement. AI tools that integrate with website platforms make this information significantly easier to access.
For example, Webflow supports a HubSpot integration that tracks website traffic. You can then use HubSpot’s AI-powered data analysis tools to extract actionable insights that’ll help you improve your site.
Upgrade your data analytics with Webflow
Webflow supports integrations and solutions that leverage AI to assist with analyzing data, writing content, and more. The platform has all the tools you need to create visually stunning, data-rich websites without writing a single line of code.
Explore Webflow’s marketing automation and analytics integrations today and get the most from your AI tools.
If Webflow is of interest and you'd like more information, please do make contact or take a look in more detail here.
Credit: Original article published here.